Developments in QuIP coding
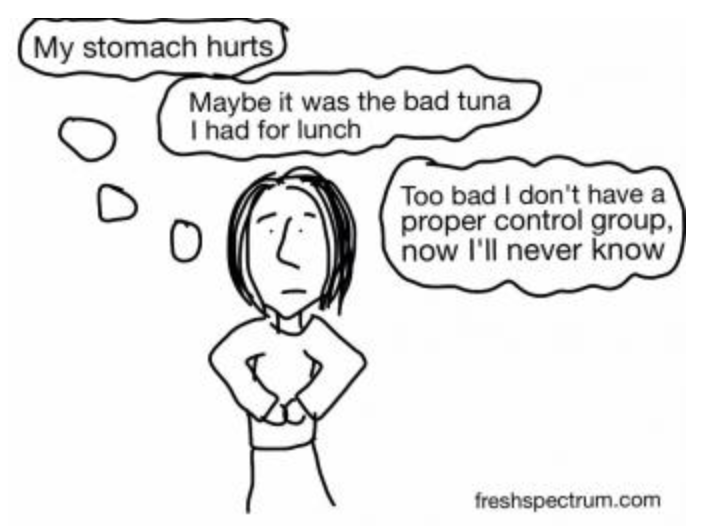
25 June 2019 | News
|We never stand still at BSDR, and the last few months have been all about reviewing the approach we take to coding our data! This short blog will summarise some of these conversations and link to a new resource for QuIP analysts to understand the implications of any changes for coding QuIP data.
The QuIP approach to coding is fundamentally about looking for stories of change and documenting causal claims in interview transcripts. We are specifically looking to document the relationships between reported cause and effect; what do respondents say caused reported changes? Summarising this data enables us to see a big picture causal network of the many variables which occur in often non-linear relationships between inputs, outputs and outcomes.
As QuIP coding developed, we focused on coding drivers and outcomes in the text, as well as allocating attribution codes. We have now moved away from defining the tags created through inductive coding as either drivers or outcomes, instead using the analysis software to determine what is a driver or an outcome based on its position in the causal chain. This gives the analyst more freedom to use the same descriptors/tags as either drivers or outcomes – depending on how different respondents document a change story. A starting point for some respondents may be a mid-point for others, so forcing one event or input to be a ‘driver’ sometimes creates an artificial distinction.
- A tag followed by any other tags in the same row of text is now automatically be considered a source, or Driver of change. It is considered to have a causal relationship with the tags following it.
- Any tag at the end of a row of tags, with nothing following it, is automatically be considered a target, or Outcome.
More information on this change, including a change to the type of tags we use, and the codes used for attribution coding – is contained in a briefing note for QuIP analysts. (Please note that this is aimed at analysts who are already familiar with QuIP coding, this is not a step-by-step guide to coding.)
In addition, we have been thinking very hard about how to code the type of relationships between cause and effect, and how to gather more information about necessity and sufficiency; what packages of ‘drivers’ and other contextual factors are either necessary or sufficient for a particular outcome to occur. For more on this, please read Rick Davies’ recent M&E News review of our book, Attributing Development Impact, where he goes into detail about some of the discussions we have had thus far on the potential to delve further into QuIP data – including using a Confusion Matrix. Rick makes the important point that it is currently not possible to use the dashboard to automatically identify “instances of ‘configurational causality’… packages of causes that must be jointly present for an outcome to occur.” This is an exercise currently communicated in narrative form by skilled analysts, using network visualisations to help evidence claims. Automating this task is a much harder nut to crack; this is not a purely theoretical or mathematical discussion about which numbers to use – but has to be considered in the context of how messy qualitative data can often be, and the extent to which we can apply mathematical formulae to semi-structured responses.
For more on this, read a recent American Evaluation Association blog – Measuring Necessity and Sufficiency by Ryan Watkins (which cites Rick Davies’ work on EvalC3).
QuIP does not use a control group to isolate differences between those who received an intervention and those who didn’t, but relies on self-reported attribution to establish causal pathways. At a theoretical level, the idea of using that data to establish whether an intervention is ‘necessary’ or ‘sufficient’ for change to occur is very appealing, but we have to be very careful how much such data is quantified and potentially taken out of context (see our paper on how to use QuIP numbers and visualisations for more). Caveats notwithstanding, we continue to explore the potential to code more efficiently, including new software (working with Steve Powell of Theory Maker). One feature would be the ability to code stories in pairs rather than chains (‘x leads to y’ and ‘y leads to z’). These pairs can then be put together to create larger network maps, whilst allowing for one pair in a chain to be negative, and another positive – reflecting the real complexity of non-linear life!
We’ll keep you updated on future developments – and look forward to more detailed discussions on coding! Join the discussion on twitter – @bathsdr
The cartoon in this blog was reproduced with the kind permission of the brilliant Chris Lysy at FreshSpectrum.com. You can subscribe to Chris Lysy’s cartoons via Patreon.
2 Comments
Hi BSDR! Following your brilliant progress with great interest!
There’s one disagreement I have with the way QuIP asks the “why question”.
I don’t think the right way to elicit information about someone’s causal map of a particular domain (e.g. in order to find out how a project influenced that domain) is to ask about changes since a certain time point.
An example of the kind of context in which the “changes” question is problematic:
> the government stopped fixing the riverbank, but NGO X intervened to keep fixing it regularly. If someone doesn’t fix the riverbank, there are more floods.
If you ask about changes, in the literal sense, there won’t be any, because the intervention served to maintain the status quo. It might be there are contrast situations like other regions where the government withdrew and no-one stepped in, or a contrast with some previous situation, but there might not be. Either way, the question about changes will only work, if it works at all, because people implicitly understand that we really want to know about the whole causal map, not just a change over time. So why not ask that directly? Why not ask, “how do things work around here vis-a-vis floods? Why are there sometimes more or fewer floods?”
BSDR has a whole wealth of experience and caveats re how to pose these questions, what works and what doesn’t as a question. So I’m hesitant to question this obviously effective way of working. But, as an evaluator I’ve often posed the alternative question, “how does X work around here?” and found it fine.
“One feature would be the ability to to code stories in pairs rather than chains.. These pairs can then be put together to create larger network maps, whilst allowing for one pair in a chain to be negative, and another positive – reflecting the real complexity of non-linear life” YES!!!